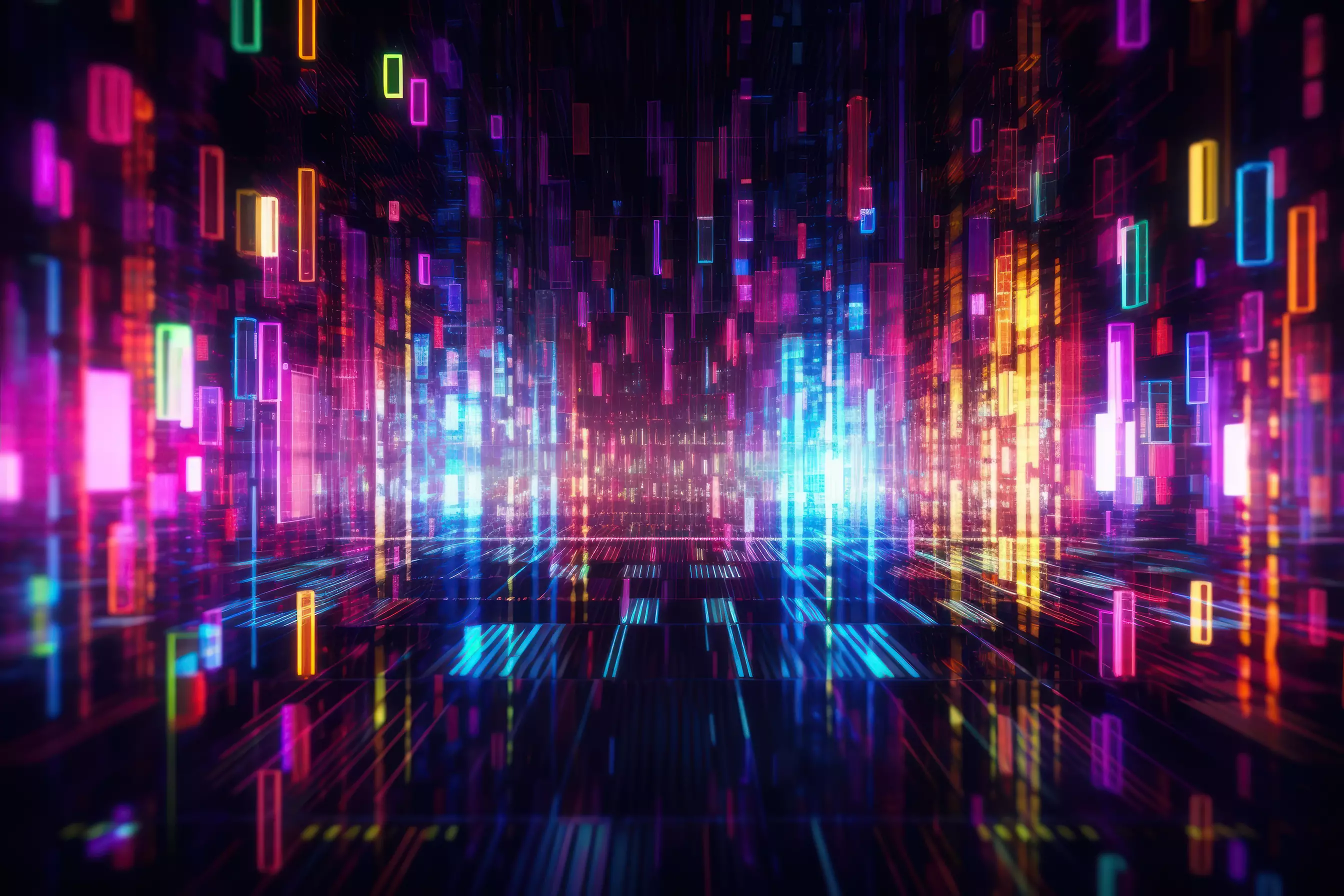
Best Practices for Architecting AI Systems
By Jeff Jenkins, ManTech AI Systems ArchitectIntro – Setting the Table
Architecture is broadly defined as the carefully designed structure of ‘something’. Whether the ‘something’ in question is a building, software or even a restaurant menu, three central concepts must be understood and applied:
- Design Principles – These lay the foundation for a creating successful Artificial Intelligence (AI) systems and ensuring their survival and evolution.
- Scalability Considerations – As requirements grow, so do the challenges of maintaining performance and efficiency.
- Deployment Strategies – Deploying AI systems requires careful planning and execution, especially when integrating with legacy systems
Design Principles
- Agile Design Applied to Architecture: Agile design principles, found in software development, can significantly enhance the architectural process of designing AI systems. Agile methodologies prioritize flexibility, iterative progress and collaboration, which are important for the dynamic nature of AI projects.
- Iterative Development: Constantly break down the AI project scope into smaller, manageable components (microservices). Develop and test these components iteratively, allowing for continuous improvement and adaptation based on feedback. This approach helps with implementation challenges discovered when testing architectures.
- Cross-functional Teams: Assemble teams with diverse expertise, including data scientists, software engineers, testers, User Interface/User Experience (UI/UX) professionals and domain experts. This collaborative foundation ensures that the AI system is designed with a holistic view, considering various perspectives and requirements.
Continuous Integration and Delivery (CI/CD)
Implement CI/CD pipelines to automate the testing and deployment of AI models and components. This approach accelerates the development process and ensures that updates are seamlessly integrated into the system.
- Robust System Integration:
AI systems often need to interact with existing third-party, Commercial Off-the-Shelf (COTS) or Government Off-the-Shelf (GOTS) software, databases and hardware. Ensuring robust system integration is fundamental for the seamless operation and scalability of AI solutions to utilize outside information and capabilities. - Modular Architecture: Design the AI system using a modular, service-oriented approach, where each component can function independently and interact with other components through well-defined interfaces. This modularity simplifies integration and allows for easier maintenance and scaling.
- Standardized Protocols and Application Programming Interfaces (APIs): Use standardized communication protocols and APIs to facilitate interaction between the AI system and other software components. Standardization reduces compatibility issues and simplifies the integration process.
- Data Management and Governance: Robust data management practices ensure the quality, consistency, and security of data used by any AI system. Implement strong data governance policies to manage data access, storage and processing, while ensuring compliance with regulatory requirements, which may differ by project and customer.
Personalized UI/UX
UI and UX are critical components of AI systems, as they directly impact user adoption and satisfaction. Personalizing the UI/UX can enhance the usability and effectiveness of AI solutions.
Here’s three ways to achieve personalized UI/UX:
- User-Centered Design: Focus on the needs and preferences of end-users throughout the design process. Conduct user research, gather feedback and iterate on the design to create intuitive and user-friendly interfaces.
- Adaptive Interfaces: Implement adaptive interfaces that can adjust based on user behavior and preferences. Use AI techniques, such as machine learning, to analyze user interactions and personalize the interface accordingly.
- Accessibility and Inclusivity: Ensure that the AI system is accessible to all users, including those with disabilities. Design with inclusivity in mind, providing features like voice commands, screen readers and customizable interface options to cater to diverse user needs.
By designing your AI systems with intention, adhering to such fundamental design principles as agile methodologies, robust systems integration and personalized UI and UX, you can ensure you’ve laid the foundation for successful Artificial Intelligence (AI) systems that are resilient, ensuring their survival as needs expand and evolve.
Scalability Considerations
Without addressing scalability from the start, AI systems may be unable to grow. Teams should therefore consider horizontal and vertical scalability considerations from day one and prepare for the heaviest use cases to gracefully accommodate growth.
Training Requirements
Training AI models, especially deep learning models, is a resource-intensive process that requires careful planning and robust infrastructure. Architecting for training
requirements involves several key considerations:
- Compute Power: Training AI models necessitates substantial computational power, often involving Graphics Processing Units (GPUs) or specialized
hardware like Tensor Processing Units (TPUs). It's essential to architect a system that can efficiently distribute and parallelize training tasks to maximize resource utilization. Cloud platforms like Amazon Web Services, Google Cloud, and Microsoft Azure offer scalable solutions, enabling dynamic allocation of resources
based on training needs. - Data Management: Effective data management is crucial for successful training. This includes ensuring data quality, preprocessing, and augmentation.
Architecting a pipeline that can handle large volumes of training data, performing necessary transformations, and feeding the data into the model efficiently. Leveraging distributed storage systems and data lakes can help manage vast datasets seamlessly. - Experimentation and Versioning: AI development is iterative, requiring continuous experimentation and model versioning. Architecting a system that supports easy experimentation, tracking of model versions, and reproducibility of results is vital.
Large Scales of Data at Runtime
Once an AI model is trained, it must be deployed to assess its ability to handle real-time data processing and inference. Architecting for runtime data handling involves
addressing several challenges:
- Scalability and Performance: The system must be designed to scale horizontally, allowing it to handle increasing volumes of data without degrading
performance. This can be achieved through microservices architecture, containerization (using Docker and Kubernetes), and load balancing. Ensuring low latency and high throughput is critical, especially for applications requiring real-time responses. - Data Ingestion and Processing: Architecting a robust data ingestion pipeline is essential for processing large-scale data at runtime. This includes integrating
with various data sources, performing real-time data transformations, and ensuring data consistency. Many open-source technologies can facilitate real- time data streaming and processing while enabling data scientists and engineers to add value. - Monitoring and Maintenance: Continuous monitoring and maintenance of the AI system is crucial to ensure its reliability and performance. Implementing observability tools can help monitor system health, track key performance metrics, and detect anomalies. Automated maintenance processes, such as rolling updates and auto-scaling, can further enhance system robustness.
Large Numbers of Users
AI systems often need to serve a large and diverse user base, necessitating an architecture that can handle high concurrency and ensure a seamless user experience:
- Load Balancing and Traffic Management: To manage high user traffic, implementing effective load balancing strategies is essential. This involves distributing incoming requests across multiple servers to ensure even load distribution and prevent bottlenecks. On-premises and cloud-based load balancers can help manage traffic efficiently.
- User Authentication and Authorization: Ensuring secure access to the AI system is paramount, especially when dealing with sensitive data. Architecting a robust authentication and authorization framework, using standards like Open Auth, JSON Web Tokens, and Security Assertion Markup Language, can help manage user access and protect against unauthorized use. Several open-source authentication management platforms can enable role and attribute-based access controls.
- Personalization and User Experience: Delivering a personalized user experience will enhance user satisfaction and engagement. Architecting the system to collect and analyze user behavior data, and using this data to tailor responses and recommendations can create a more intuitive and effective user experience. Leveraging AI techniques like collaborative filtering and content- based filtering can aid in personalizing interactions.
Balancing performance and efficiency is key when designing AI systems, as is ensuring they are robust and flexible enough to scale effectively as needs evolve.
Deployment Strategies
Architecting AI systems at scale involves making strategic decisions about deployment strategies that align with business goals, technical requirements, and customer needs.
Working with Existing Customer Assets in Their Own Environment
When customers have established infrastructure and data assets, deploying AI systems within their existing environment is a practical and efficient strategy. This approach leverages the customer’s current investments in hardware, software, and data storage, minimizing disruption and reducing the need for extensive reconfiguration.
When to Exercise This Strategy:
- Data Sensitivity and Compliance: If the customer’s data is highly sensitive or subject to strict regulatory requirements, keeping the AI system within their environment helps to ensure compliance and data security.
- Infrastructure Investment: Customers who have already invested significantly in their infrastructure may prefer to maximize the return on their investment by integrating AI capabilities without major changes.
- Operational Continuity: For businesses that require minimal downtime and disruption, deploying AI within the existing environment allows for a smoother transition and continuous operations.
Migrating Existing Customer Assets to Other Environments
In some cases, migrating customer assets to a new environment, such as a cloud platform, can offer significant benefits in terms of scalability, flexibility, and access to advanced AI tools and services. This strategy involves transferring data, applications, and workloads to a new infrastructure that better supports AI initiatives.
When to Exercise This Strategy:
- Scalability Needs: If the customer’s existing environment lacks the scalability required for AI workloads, migrating to a cloud platform can provide the necessary resources and elasticity.
- Cost Efficiency: Cloud environments often offer cost-effective solutions for AI deployments, with pay-as-you-go models and reduced overhead for hardware maintenance.
- Access to Advanced Tools: Cloud platforms provide access to cutting-edge AI tools, frameworks, and services that may not be available or feasible to implement in the customer’s current environment.
Starting from Scratch
For businesses embarking on new AI projects without existing infrastructure constraints, starting from scratch presents an opportunity to design and build AI systems tailored to specific needs and goals.
When to Exercise This Strategy:
- Research and Development Projects: New initiatives without legacy systems or data can benefit from a fresh start, enabling the use of the latest technologies and best practices in AI development and deployment.
- Custom Requirements: When the AI system requires highly specialized configurations or integrations, starting from scratch allows for a bespoke solution that meets precise specifications.
- Innovation and Experimentation: For organizations looking to innovate and experiment with AI, building a new environment provides the flexibility to explore different approaches and iterate rapidly.
Final Thoughts
Architecting AI systems for success requires a thoughtful approach to design, scalability, and deployment.
Executing successful design involves following agile design principles, ensuring robust system integration, and creating personalized UI/UX use cases for a wide variety of users. When this is achieved, organizations are enabled to build scalable and effective AI solutions that meet the needs of their users and drive business value.
Architecting AI systems with different kinds of scale involves focusing on specific model training requirements, runtime data handling, and managing large user groups, organizations have a blueprint to build and deploy robust, scalable AI systems.
Whether working with existing customer assets, migrating to new environments, or starting from scratch, each approach has its unique advantages and considerations. By carefully evaluating the specific needs and constraints of each project, businesses can make informed decisions that pave the way for successful AI deployments and unlock the full potential of AI technologies.
By addressing these three central concepts, organizations can build scalable AI systems that not only meet current requirements but are also designed for future success.
Learn More
Jeff Jenkins, ManTech AI Systems Architect
To learn more about ManTech’s expertise in operationalizing Data and AI for federal agencies, contact Jeffrey Jenkins via AI@ManTech.com
Or visit our site here.